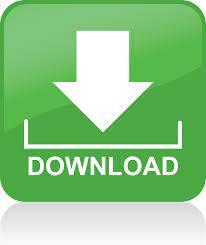
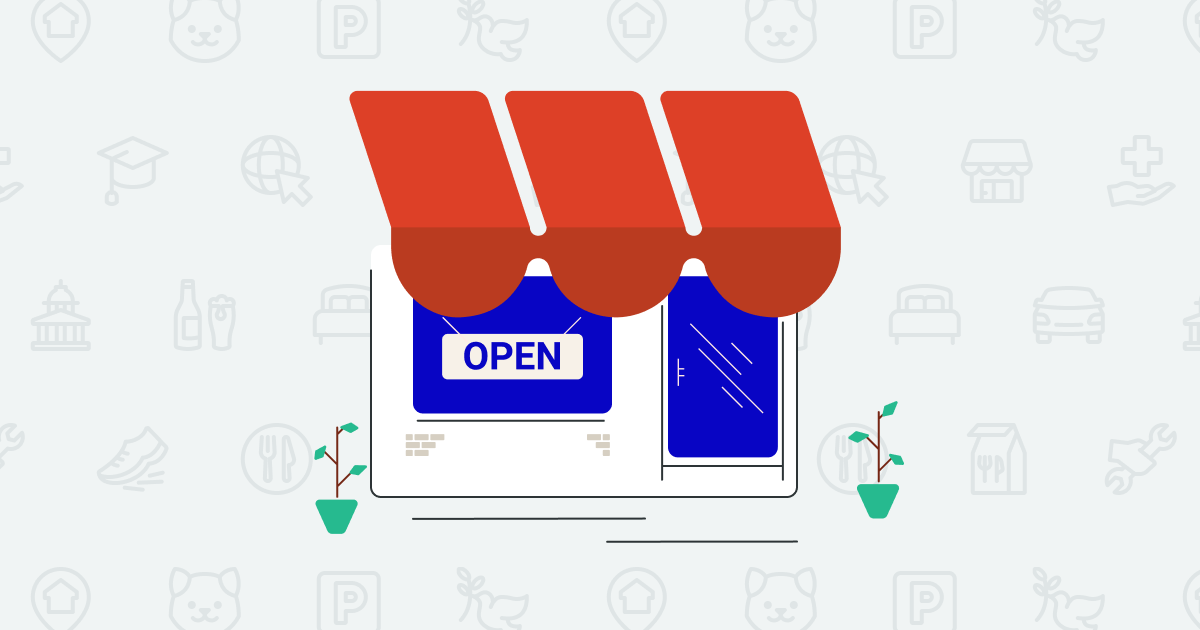
- #Delaware imaging network how to#
- #Delaware imaging network generator#
- #Delaware imaging network code#
CycleGAN should only be used with great care and calibration in domains where critical decisions are to be taken based on its output.
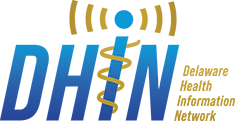
" and the predictions, thought plausible, may largely differ from the ground truth. Its outputs are predictions of "what might it look like if.
#Delaware imaging network how to#
How to interpret CycleGAN results: CycleGAN, as well as any GAN-based method, is fundamentally hallucinating part of the content it creates. Search CycleGAN in Twitter for more applications. Here we highlight a few of the many compelling examples.
#Delaware imaging network code#
Researchers, developers and artists have tried our code on various image manipulation and artistic creatiion tasks. Nice explanation by Hardik Bansal and Archit Rathore, with Tensorflow code documentation. Other ImplementationsĮxpository Articles and Videos Two minute papers Please contact the instructor if you would like to adopt this assignment in your course. Roger Grosse for "Intro to Neural Networks and Machine Learning" at University of Toronto. If you have questions about our PyTorch code, please check out model training/test tips andĬycleGAN course assignment code and handout designed by Prof. "Unpaired Image-to-Image Translation using Cycle-Consistent Adversarial Networks", in IEEE International Conference on Computer Vision (ICCV), 2017. Jun-Yan Zhu*, Taesung Park*, Phillip Isola, and Alexei A.

Quantitative comparisons against several prior methods demonstrate the superiority of our approach. Qualitative results are presented on several tasks where paired training data does not exist, including collection style transfer, object transfiguration, season transfer, photo enhancement, etc. Because this mapping is highly under-constrained, we couple it with an inverse mapping F: Y → X and introduce a cycle consistency loss to push F(G(X)) ≈ X (and vice versa). Our goal is to learn a mapping G: X → Y, such that the distribution of images from G(X) is indistinguishable from the distribution Y using an adversarial loss. We present an approach for learning to translate an image from a source domain X to a target domain Y in the absence of paired examples. However, for many tasks, paired training data will not be available. The results of the experiments show that FPGAN outperforms the baseline methods.Image-to-image translation is a class of vision and graphics problems where the goal is to learn the mapping between an input image and an output image using a training set of aligned image pairs. Finally, we tested our model and four other methods on the CelebA, MORPH, RaFD, and FBDe datasets. This protocol can be used for the evaluation of face de-identification and privacy protection. Moreover, we proposed a new face de-identification evaluation protocol to check the performance of the model. In our experiments, we applied FPGAN to face de-identification in social robots and analyzed the related conditions that could affect the model. Subsequently, we propose the pixel loss, content loss, adversarial loss functions and optimization strategy to guarantee the performance of FPGAN.

#Delaware imaging network generator#
Then, a generator with an improved U-Net is used to enhance the quality of the generated image, and two discriminators with a seven-layer network architecture are designed to strengthen the feature extraction ability of FPGAN. First, we propose FPGAN and mathematically prove its convergence. In this paper, we propose a new face de-identification method based on generative adversarial network (GAN) to protect visual facial privacy, which is an end-to-end method (herein, FPGAN).
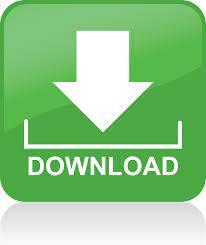